Artificial Intelligence has been making significant strides in the field of archeology. Here are a few ways in which AI is revolutionizing the discipline:
Remote sensing: AI-powered remote sensing techniques can analyze vast amounts of satellite imagery and other geospatial data to identify potential archaeological sites and features.
Pattern recognition: AI algorithms can help archaeologists identify patterns in data that might not be apparent to the human eye, aiding in the interpretation of artifacts and the reconstruction of past societies.
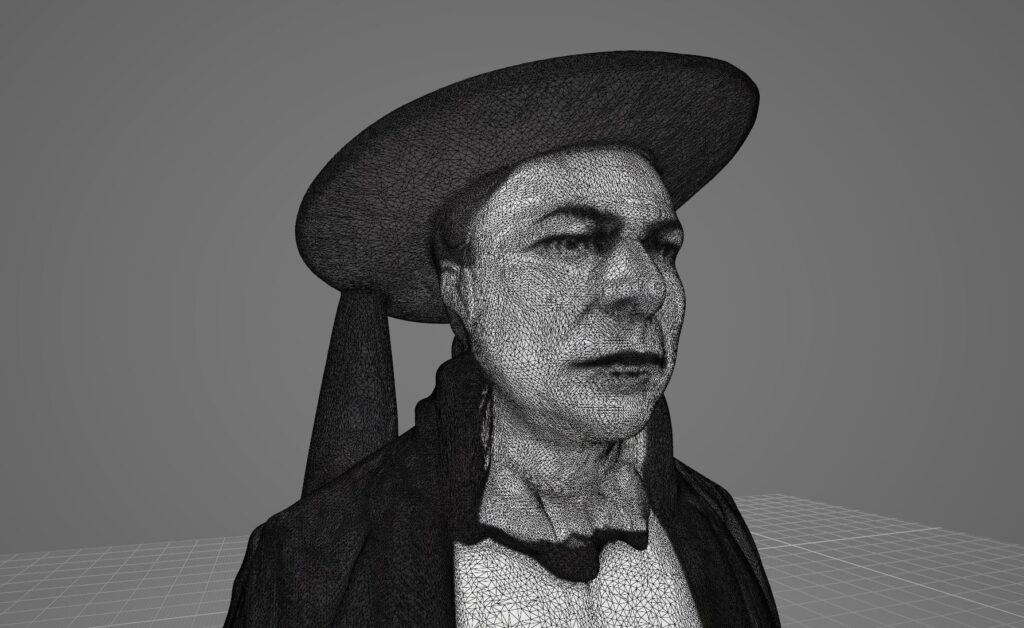
Virtual reconstruction: AI can be used to create 3D models of archaeological sites and artifacts, allowing researchers to visualize and study them in greater detail.
Data analysis: With the help of AI, archaeologists can analyze large datasets more efficiently, uncovering new insights and making connections that might otherwise be missed.
Preservation: AI-powered technologies, such as digital scanning and 3D printing, can help preserve fragile artifacts and sites for future generations.
As AI continues to advance, its applications in archaeology will likely become even more widespread and sophisticated, unlocking new discoveries and deepening our understanding of the past.
Forplay : animating Zacharie le Rouzic
Zacharie Le Rouzic, born on December 24, 1864, in Carnac and passing away on December 15, 1939, was a pioneering French archaeologist and prehistorian in the field of megalithic archaeology in Morbihan. Despite his humble beginnings, Le Rouzic made significant contributions to the study and understanding of megalithic structures in the region, leaving a lasting legacy in the field of archaeology.
Working from a photo
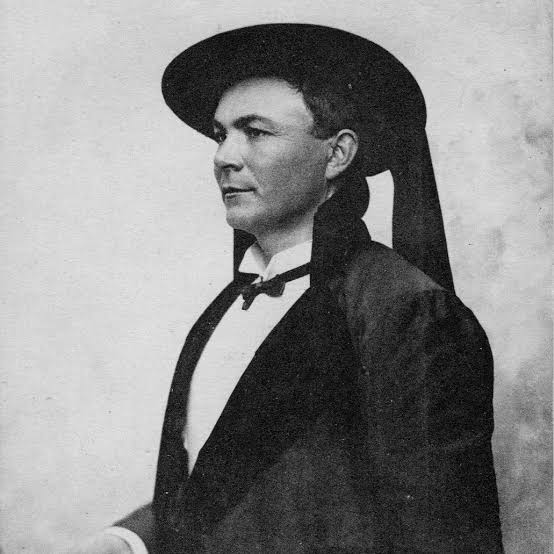
Colourising
Many platforms allows to colourise an old photograph. Coloring a black and white photo using AI typically involves a deep learning model that has been trained on a large dataset of color images. Here’s a simplified explanation of how the process works:
Input: The black and white photo is fed into the AI model as an input.
Feature extraction: The model analyzes the input image and identifies various features, such as edges, shapes, and textures.
Mapping features to colors: Based on the extracted features and the patterns it has learned from the training data, the model predicts the most probable colors for each pixel in the image.
Output: The model generates a colorized version of the input image, with each pixel now assigned a color value.
It’s important to note that the colors generated by the AI model might not always be historically accurate, as it relies on patterns learned from modern color images and makes predictions based on those patterns. Nonetheless, AI-powered colorization can provide a fascinating glimpse into the past by adding a sense of realism and vibrancy to historical photographs.
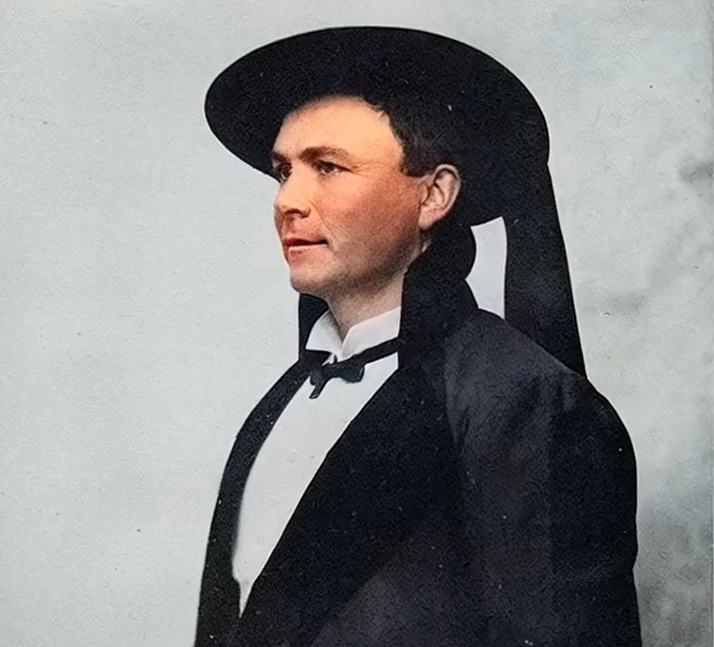
Animating the image
Animating a photo using AI typically involves a deep learning technique called « generative adversarial networks » (GANs). This process can be broken down into several steps:
Training data: First, the AI model is trained on a large dataset containing both photos and corresponding videos, allowing it to learn how to generate realistic video frames based on a single image.
Input image: A still photo is provided as input to the AI model.
Feature extraction: The model analyzes the input image to identify key features, such as the subject’s pose, facial expressions, and environmental elements.
Motion estimation: Based on the extracted features, the AI model estimates the motion of the subject and the background elements in the image.
Frame generation: Using the estimated motion information, the AI model generates a series of new video frames that create the illusion of movement. This can include animating the subject’s facial expressions, hair movement, or changes in the environment, like moving clouds or flowing water.
Output: The model produces an animated sequence based on the original still photo.
While AI-generated animations are not always perfect and may exhibit some artifacts or inconsistencies, they showcase the potential of machine learning techniques to create engaging and dynamic visual content from static images.
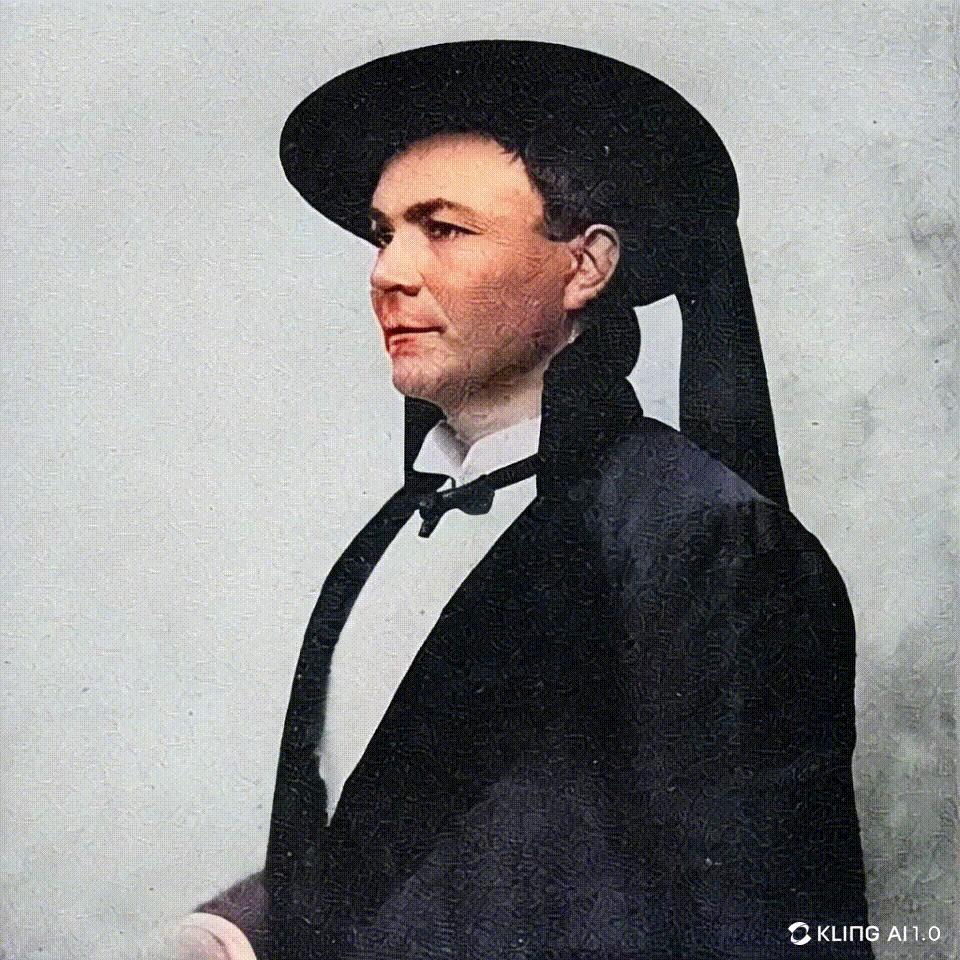
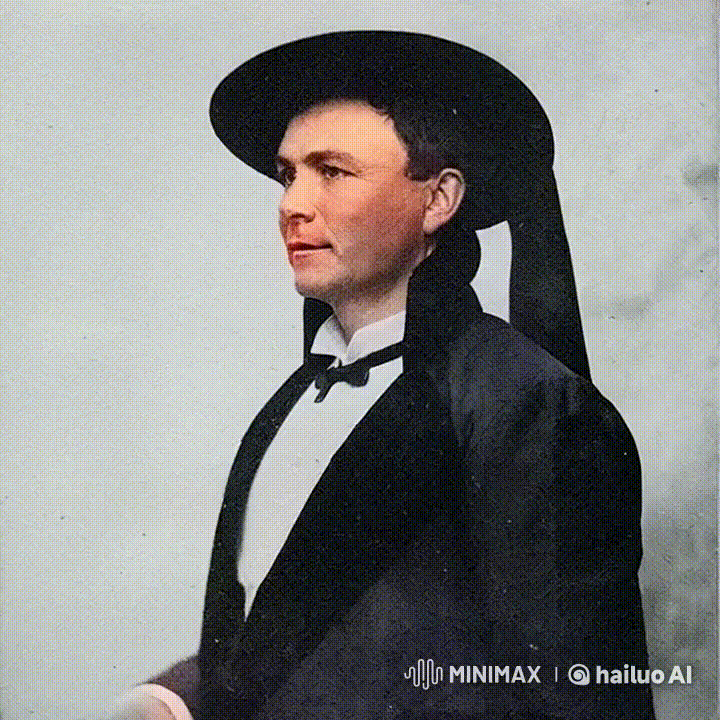
From photo to 3D
Converting a single photo into a 3D representation using AI typically involves a process called « monocular depth estimation. » This method allows the AI model to estimate the distance between different objects in the scene and create a 3D reconstruction based on that information. Here’s how it works:
Input image: A single 2D image is provided as input to the AI model.
Feature extraction: The model analyzes the input image to identify various visual cues, such as edges, textures, and perspective distortion, which can provide clues about the 3D structure of the scene.
Depth map estimation: Based on the extracted features and patterns learned from a large dataset of 2D images and their corresponding depth maps, the AI model generates a depth map for the input image. The depth map assigns a depth value to each pixel in the image, representing its distance from the camera.
3D reconstruction: Using the estimated depth map, the AI model reconstructs the 3D structure of the scene. This can involve creating a point cloud or a mesh representation of the objects in the image, which can be further refined and textured to create a realistic 3D model.
Output: The AI model generates a 3D reconstruction of the scene, which can be viewed from different angles and potentially integrated into virtual or augmented reality applications.
While monocular depth estimation is a powerful technique, it has some limitations, such as ambiguity in areas with limited visual cues or textureless surfaces. However, ongoing research and advancements in AI technology continue to improve the accuracy and quality of single-image 3D reconstruction.
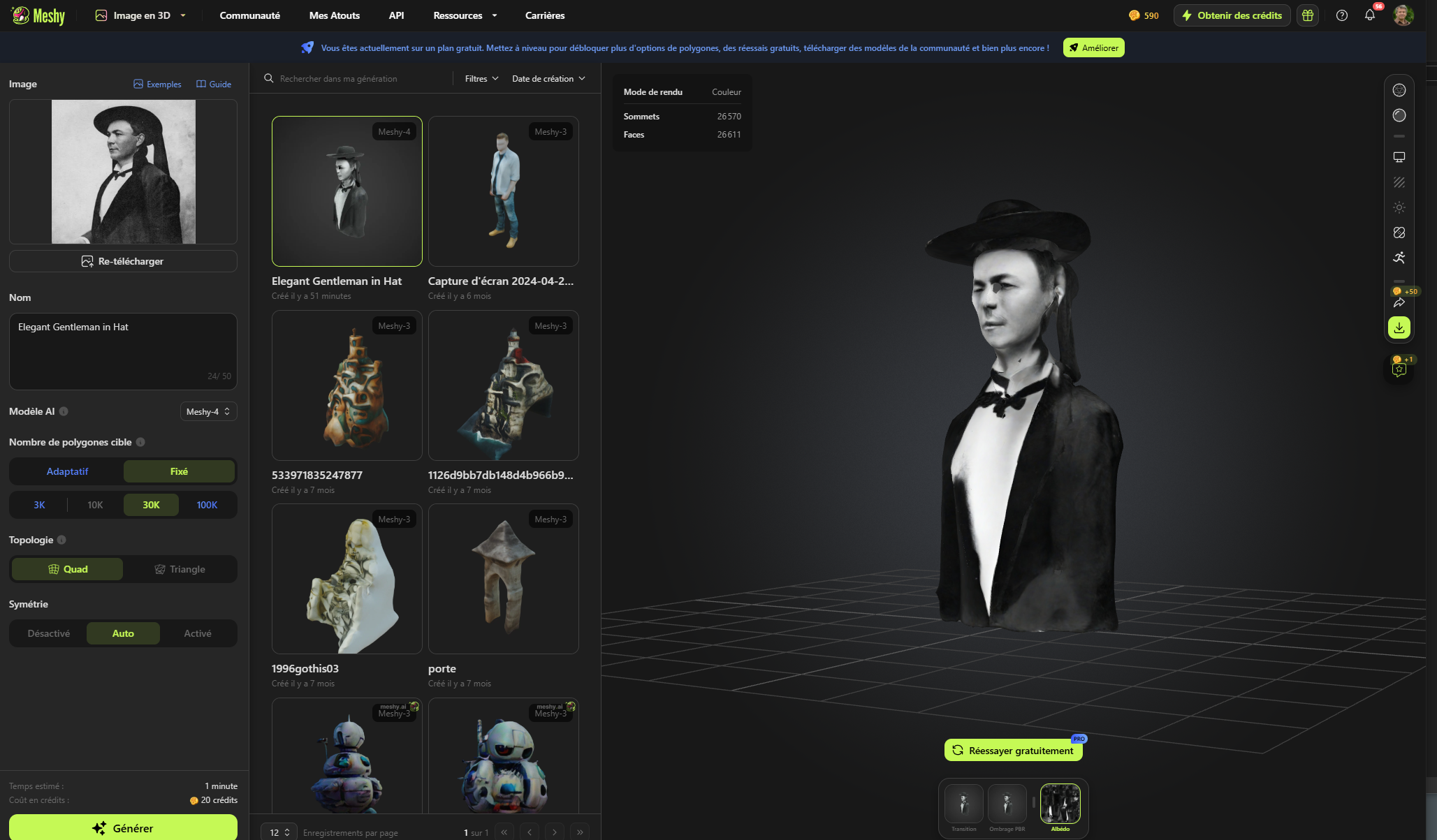
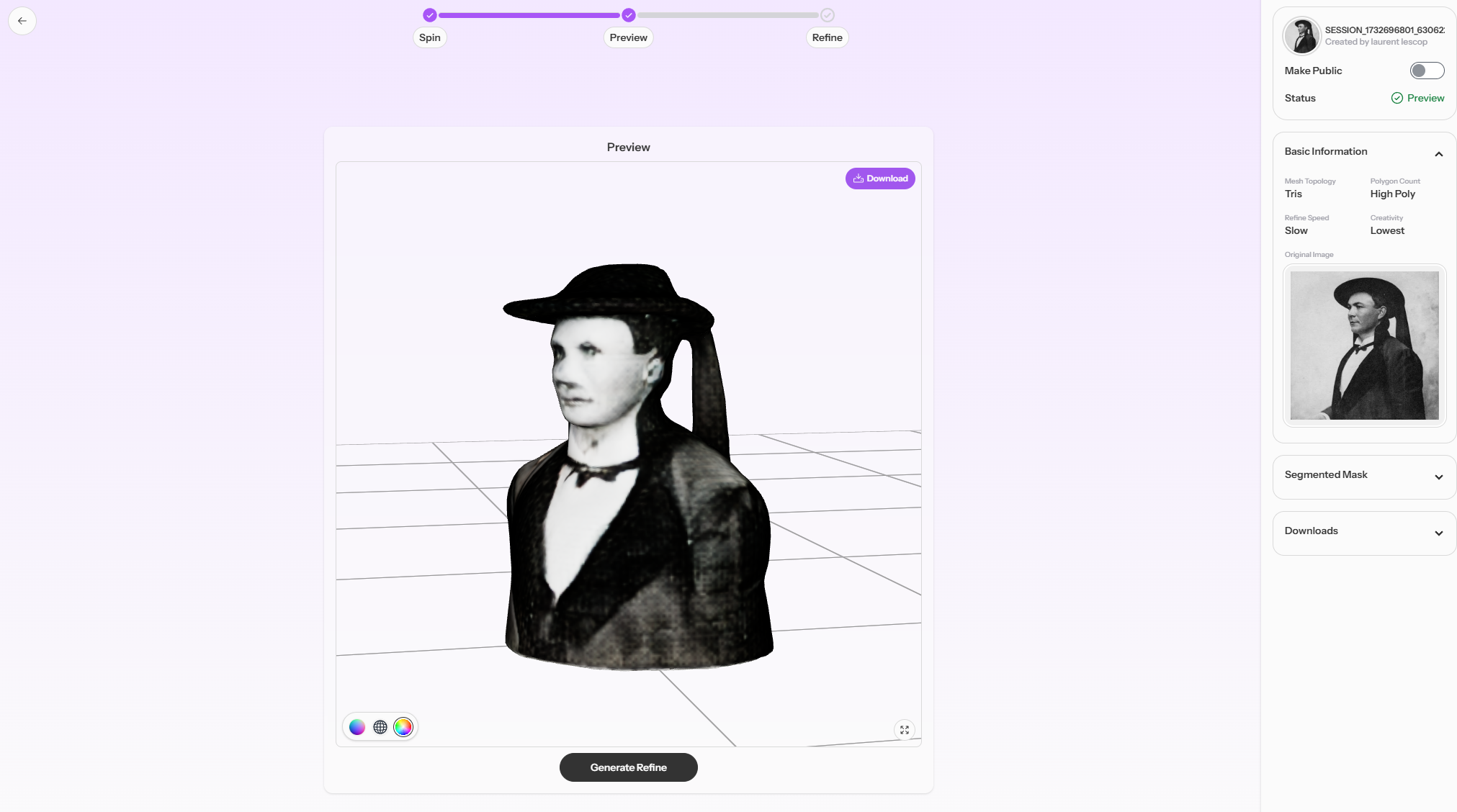
The best result comes with Tripo
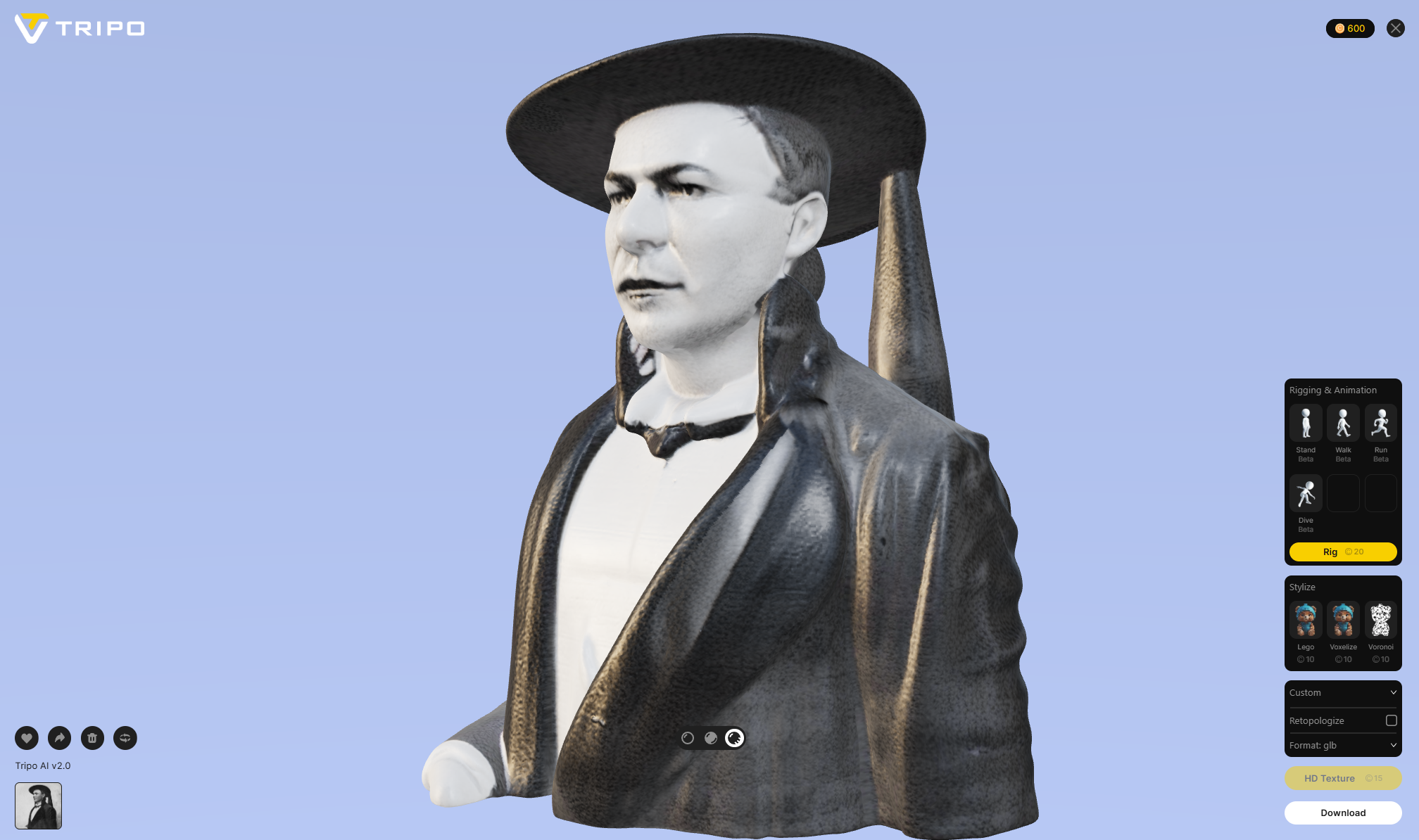
Conclusion
In conclusion, the exploration of these AI-driven techniques – colorization, animation, and 3D reconstruction – demonstrates the incredible potential of artificial intelligence to enhance our experience and understanding of visual media. By leveraging deep learning models trained on vast datasets, AI can extract valuable information from still images and create compelling, dynamic, and immersive content.
Colorization allows us to breathe new life into historical black and white photos, offering a glimpse into the past with added depth and realism. AI-powered animation enables us to animate still images, creating engaging visual stories from single frames. Finally, monocular depth estimation empowers us to transform 2D images into 3D representations, unlocking new possibilities for interaction and exploration in virtual and augmented reality environments.
While these techniques continue to evolve and improve, they already demonstrate the transformative impact of AI on visual media, expanding the boundaries of creativity and enriching our understanding of the world around us. As AI technology progresses, we can expect even more innovative applications and advancements in the realm of image processing and generation.